Introduction
The landscape of artificial intelligence (AI) is rapidly evolving, driven by the insatiable hunger for data that fuels machine learning models.Yet, recent studies have unveiled a troubling trend: the data essential for training these intelligent systems is becoming increasingly scarce.This article delves into the implications of this phenomenon, exploring the causes, consequences, and potential solutions to the challenges faced in acquiring quality AI training data.The Importance of Quality Data in AI
The success of any machine learning model hinges on the quality and quantity of its training data.These algorithms learn by identifying patterns and making predictions based on the data they are fed.The relationship between data and AI performance is straightforward: more data often leads to better results.However, as the demand for high-quality datasets intensifies, researchers and developers are facing significant hurdles in obtaining the necessary information.Why Is Training Data Disappearing?
Several factors contribute to the dwindling availability of training data for AI: 1. **Data Privacy Regulations**: Governments and organizations are increasingly implementing strict data privacy laws, such as the General Data Protection Regulation (GDPR) in Europe.These regulations limit the types of data that can be collected and shared, putting a strain on the repositories firms traditionally relied upon. 2. **Data Cost and Ownership Issues**: Acquiring data can be prohibitively expensive, particularly for small enterprises or startups.Additionally, many companies are less willing to share their data due to fears of losing competitive advantages, leading to the fragmentation of data sources. 3. **Underrepresentation of Certain Groups**: Data can often be skewed or biased, making it less useful for training AI systems.For instance, datasets that lack diversity might lead to models that perform poorly when faced with real-world applications.The Consequences of Data Scarcity
The decline of quality training data presents several serious challenges for AI development: 1. **Stagnation of AI Innovation**: As data becomes harder to find, AI researchers may struggle to advance their models.The inability to train on extensive datasets can stifle innovations and limit the development of new applications. 2. **Increased Costs for AI Development**: Companies may have to invest significantly more resources to create their datasets or rely on expensive third-party solutions to procure what they need. 3. **Ethical Dilemmas**: As developers search for new data sources, ethical concerns might arise, particularly if data is sourced from less transparent channels.The use of poorly sourced information may exacerbate biases and lead to AI systems that reflect and perpetuate societal inequalities.Overcoming Training Data Challenges
While the issues surrounding data availability are daunting, there are strategies that can be employed to tackle this growing concern:1. Transfer Learning
Transfer learning involves taking knowledge gained from one task and applying it to a different but related task.By leveraging pre-trained models, organizations can utilize less data for specific applications, significantly reducing the need for extensive datasets.2. Data Augmentation
Data augmentation techniques can artificially increase the size of datasets by creating variations of existing data points.For example, in image processing, small modifications, such as rotations or color changes, can provide models with more training examples, helping prevent overfitting.3. Generative Adversarial Networks (GANs)
GANs can create realistic synthetic data by training two neural networks against each other: one generates data, while the other evaluates it.This approach can produce vast amounts of data for training purposes without infringing on privacy issues.4. Collaboration and Data Sharing Agreements
Researchers and companies can benefit from forming partnerships and data-sharing agreements.Pooling resources can help organizations gain access to a wider variety of datasets while ensuring that privacy regulations are respected.Conclusion
The finding that training data for AI is disappearing fast signals a critical moment for the industry.As technology continues to evolve and the demand for intelligent systems surges, addressing data scarcity is paramount.By embracing innovative techniques such as transfer learning, data augmentation, and collaborative approaches, the AI community can navigate this challenge and continue to drive forward one of the most revolutionary technologies of our era.References
Gretton, A., Borgwardt, K. M., Rasch, M., et al. (2007). A kernel method for the two-sample-problem.Advances in neural information processing systems, 513-520. Zhang, Y., & Yang, Q. (2010). A survey on transfer learning.IEEE Transactions on knowledge and data engineering, 22(10), 1345-1359. Trask, A. W. (2022). Grokking Deep Learning. Kochmar, E. (2019). Getting Started with Natural Language Processing.Stay Updated with Our Newsletter
Sign up to receive the latest articles, insights, and trends.
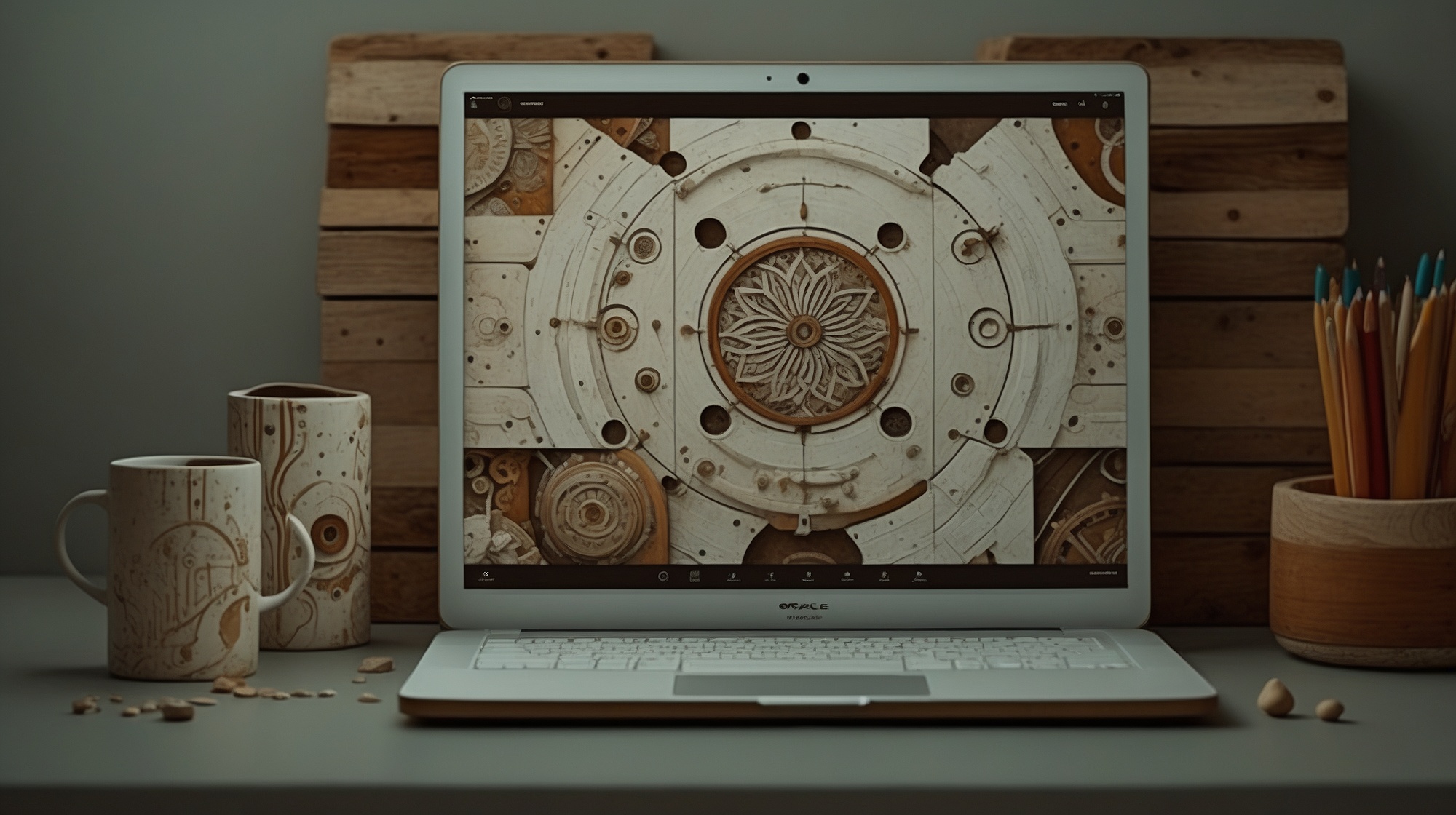