Imagine a world where finding a cure for diseases isn't a long, drawn-out process but something that can happen rapidly, thanks to technology. Welcome to the world of AI-powered drug discovery. For decades, the process of discovering new drugs has been slow, expensive, and fraught with trial and error. But now, with the advent of artificial intelligence (AI), the pharmaceutical industry is on the cusp of a revolution. AI is transforming the way drugs are discovered, developed, and brought to market, making the entire process faster, cheaper, and more efficient. Let's dive into how AI is reshaping pharmaceutical research and what it means for the future of medicine.
The Traditional Drug Discovery Process
Before we explore how AI is changing the game, it's essential to understand how drug discovery traditionally works. The process typically involves several stages:
- Target Identification: Scientists identify a biological target, such as a protein, that is associated with a disease.
- Lead Discovery: Researchers find compounds that can interact with the target.
- Preclinical Testing: The lead compounds are tested in the lab and on animals to evaluate their safety and efficacy.
- Clinical Trials: If preclinical testing is successful, the drugs are tested on humans in multiple phases to ensure they are safe and effective.
- Regulatory Approval: The drug is submitted for approval by regulatory bodies like the FDA.
- Market Launch: Once approved, the drug is manufactured and made available to patients.
This process can take over a decade and cost billions of dollars, with no guarantee of success. Many promising compounds fail at various stages, leading to significant financial losses and delays in bringing new treatments to patients.
Enter AI: Transforming Drug Discovery
AI is changing this landscape by streamlining and enhancing every step of the drug discovery process. Here’s how:
Target Identification
AI algorithms can analyze vast amounts of biological data to identify potential drug targets. By sifting through genetic information, scientific literature, and other datasets, AI can pinpoint proteins or genes associated with diseases more quickly and accurately than traditional methods. For example, deep learning algorithms can analyze gene expression data to identify biomarkers for diseases like cancer.
Lead Discovery
Once a target is identified, the next step is finding compounds that can interact with it. AI excels in this area by predicting how different molecules will behave. Machine learning models can analyze the chemical properties of millions of compounds to identify those that are most likely to bind to the target effectively. This process, known as virtual screening, significantly speeds up the identification of potential drug candidates.
AI can also generate novel compounds. Using generative models, AI can design new molecules with desired properties, creating entirely new classes of drugs that might not have been discovered through traditional methods.
Preclinical Testing
AI is revolutionizing preclinical testing by predicting how compounds will behave in biological systems. Machine learning models can simulate how drugs will interact with proteins, cells, and tissues, reducing the need for extensive laboratory experiments. This approach not only accelerates the testing process but also helps identify potential side effects early on, improving the safety profile of new drugs.
Clinical Trials
Clinical trials are notoriously time-consuming and expensive. AI can optimize this process by identifying suitable candidates for trials, predicting outcomes, and monitoring patients in real-time. Natural language processing (NLP) algorithms can analyze patient records and medical literature to match patients with appropriate trials, ensuring a diverse and representative sample.
Moreover, AI can predict which patients are most likely to respond positively to a new treatment, allowing for more targeted and efficient trials. This personalized approach increases the chances of success and reduces the time and cost of bringing new drugs to market.
Regulatory Approval and Beyond
Regulatory bodies require extensive documentation and evidence of a drug’s safety and efficacy. AI can assist in this process by automating the analysis of trial data and generating comprehensive reports. This speeds up the approval process and ensures that no critical information is overlooked.
Once a drug is approved, AI continues to play a role in monitoring its performance. By analyzing real-world data from electronic health records and other sources, AI can track the effectiveness of the drug, identify any long-term side effects, and suggest improvements or additional uses for the medication.
Real-World Examples of AI in Drug Discovery
Several AI-powered platforms and collaborations are already making significant strides in drug discovery:
Insilico Medicine
Insilico Medicine uses AI to accelerate drug discovery and development. Their AI platform can identify new drug targets, design novel molecules, and predict the outcomes of clinical trials. In one notable case, Insilico’s AI designed a new drug in just 21 days, a process that typically takes months or even years.
Atomwise
Atomwise uses deep learning algorithms to predict how small molecules will interact with target proteins. Their AI technology has been used to identify potential treatments for diseases like Ebola and multiple sclerosis. By partnering with research institutions and pharmaceutical companies, Atomwise aims to bring new drugs to market faster and more efficiently.
BenevolentAI
BenevolentAI combines machine learning with scientific expertise to discover new drugs and develop treatments for various diseases. Their AI platform analyzes vast amounts of biomedical data to identify new drug targets and predict the success of potential treatments. BenevolentAI’s technology has been used to identify potential treatments for conditions such as amyotrophic lateral sclerosis (ALS) and sarcopenia.
DeepMind’s AlphaFold
DeepMind’s AlphaFold has made headlines for its groundbreaking ability to predict protein structures with high accuracy. Understanding protein structures is crucial for drug discovery, as it allows scientists to see how drugs will interact with their targets. AlphaFold’s predictions can significantly accelerate the identification of new drug targets and the design of effective treatments.
The Benefits of AI-Powered Drug Discovery
AI-powered drug discovery offers several advantages over traditional methods:
Speed
AI accelerates every stage of the drug discovery process, from target identification to clinical trials. By automating data analysis and leveraging predictive models, AI can shorten the time it takes to bring new drugs to market. This rapid pace is especially critical in responding to emerging health threats, such as pandemics.
Cost-Effectiveness
Traditional drug discovery is expensive, with costs often exceeding billions of dollars. AI reduces these costs by streamlining research, minimizing the need for extensive laboratory experiments, and increasing the efficiency of clinical trials. The savings can be reinvested in further research and development, leading to more innovations.
Precision and Personalization
AI enables the development of personalized medicine by analyzing genetic and clinical data to tailor treatments to individual patients. This approach improves the efficacy of drugs and reduces the risk of adverse reactions. Personalized medicine is particularly valuable in oncology, where treatments can be customized based on the genetic profile of a patient’s tumor.
Enhanced Safety
By predicting potential side effects early in the development process, AI improves the safety profile of new drugs. Machine learning models can simulate how drugs will interact with biological systems, identifying toxicities and other issues before they reach clinical trials. This proactive approach reduces the likelihood of failures and ensures that only the safest drugs reach patients.
Challenges and Considerations
While AI-powered drug discovery holds immense promise, it is not without challenges:
Data Quality and Availability
AI algorithms require large amounts of high-quality data to function effectively. In the pharmaceutical industry, data can be fragmented, incomplete, or inconsistent. Ensuring data quality and integrating diverse datasets are critical for the success of AI in drug discovery.
Interpretability and Transparency
AI models, particularly deep learning algorithms, can be complex and difficult to interpret. Regulatory bodies and researchers need to understand how AI makes decisions to ensure safety and efficacy. Developing transparent and interpretable AI models is essential for gaining trust and regulatory approval.
Ethical and Legal Issues
The use of AI in drug discovery raises ethical and legal questions, such as data privacy, intellectual property rights, and the potential for bias in AI algorithms. Addressing these issues requires collaboration between technologists, ethicists, regulators, and legal experts to develop robust guidelines and frameworks.
Integration with Traditional Methods
AI is not a replacement for traditional drug discovery methods but a complement to them. Integrating AI with existing research workflows and ensuring collaboration between AI experts and biomedical scientists are crucial for maximizing the benefits of AI.
The Future of AI in Drug Discovery
The future of AI-powered drug discovery is bright, with ongoing advancements promising to further revolutionize the field:
Integration of Multi-Omics Data
Multi-omics refers to the integration of various biological data types, such as genomics, proteomics, and metabolomics. AI can analyze multi-omics data to gain a comprehensive understanding of diseases and identify novel drug targets. This holistic approach has the potential to uncover new insights and accelerate drug discovery.
AI-Driven Drug Repurposing
AI can identify new uses for existing drugs, a process known as drug repurposing. By analyzing clinical data and scientific literature, AI can uncover previously unrecognized connections between drugs and diseases. Drug repurposing is a cost-effective and time-efficient way to bring new treatments to patients.
Collaboration and Open Innovation
Collaboration between pharmaceutical companies, research institutions, and AI startups is driving innovation in drug discovery. Open innovation platforms, where researchers share data and insights, are fostering collaboration and accelerating progress. These partnerships are essential for leveraging the full potential of AI.
Advanced AI Models
The development of more advanced AI models, such as generative adversarial networks (GANs) and reinforcement learning, will further enhance drug discovery. GANs can generate novel compounds with desired properties, while reinforcement learning can optimize drug design by exploring vast chemical spaces. These cutting-edge models will continue to push the boundaries of what AI can achieve.
AI and Quantum Computing
Quantum computing holds the potential to revolutionize drug discovery by solving complex problems that are beyond the capabilities of classical computers. AI-powered quantum simulations can accelerate the discovery of new compounds and predict their interactions with biological targets. While still in its early stages, the combination of AI and quantum computing represents an exciting frontier for pharmaceutical research.
Conclusion
AI-powered drug discovery is transforming the pharmaceutical industry, offering unprecedented speed, precision, and cost-effectiveness. By leveraging the power of AI, researchers can identify new drug targets, design novel compounds, and optimize clinical trials more efficiently than ever before. The benefits of AI in drug discovery are vast, from personalized medicine to enhanced safety and beyond.
However, realizing the full potential of AI requires addressing challenges related to data quality, interpretability, ethics, and integration with traditional methods. Collaboration between technologists, scientists, regulators, and ethicists is crucial for developing robust and trustworthy AI solutions.
The future of AI in drug discovery is promising, with advancements in multi-omics data integration, drug repurposing, advanced AI models, and quantum computing poised to drive further innovation. As we continue to explore the capabilities of AI, the possibilities for revolutionizing pharmaceutical research and improving patient outcomes are limitless.
For young people interested in the intersection of technology and healthcare, AI-powered drug discovery offers an exciting and impactful career path. By staying informed, developing relevant skills, and embracing innovation, you can play a vital role in shaping the future of medicine and making a difference in the lives of patients worldwide. The journey of AI in drug discovery is just beginning, and the potential for positive change is immense. So, stay curious, keep learning, and be a part of this groundbreaking revolution.
Stay Updated with Our Newsletter
Sign up to receive the latest articles, insights, and trends.
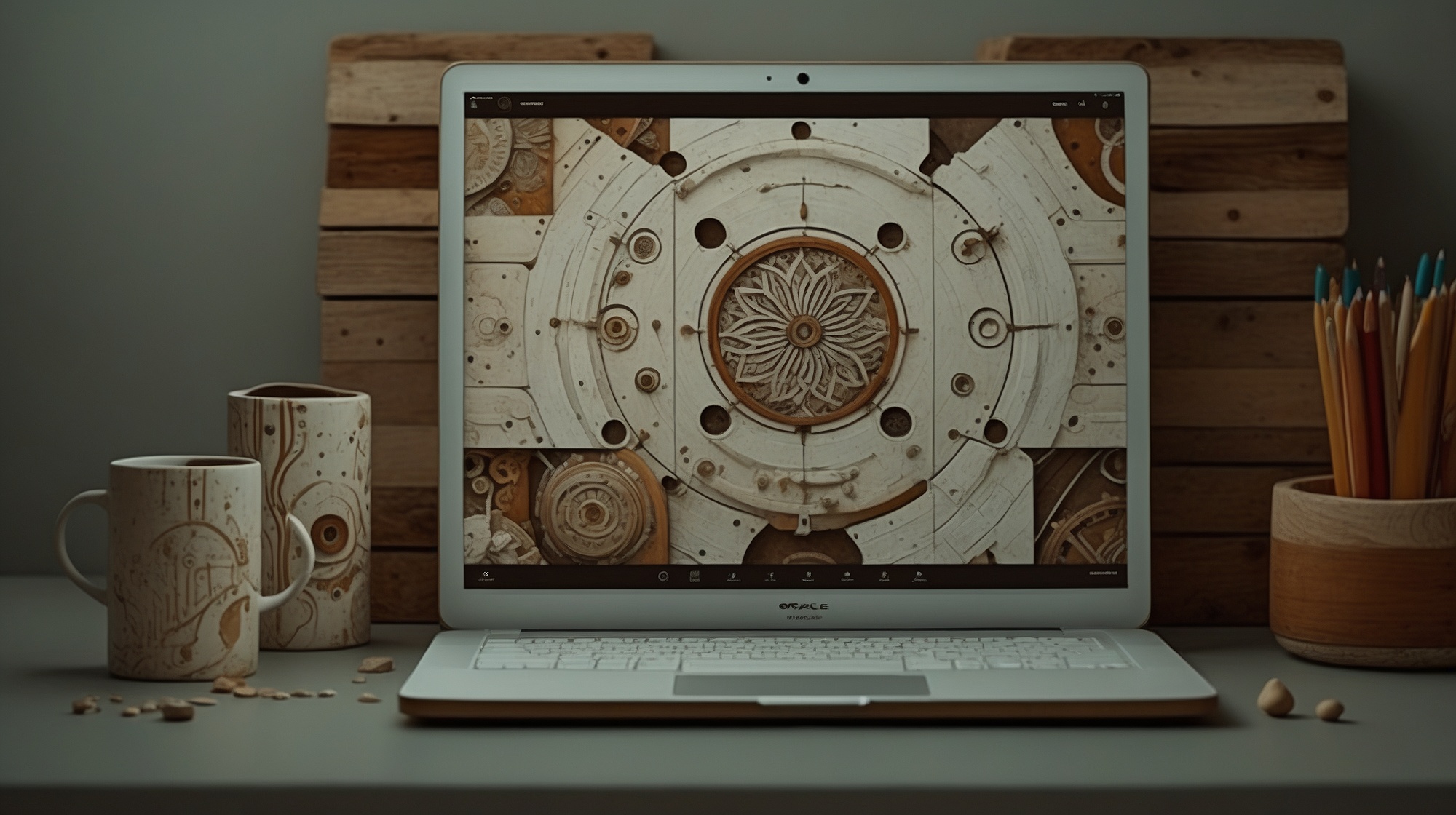